Case Study - Early detection of emission anomalies, machine failure
Collaborated on a digital twin for anomaly detection and predictive maintenance, Identified early machine failure to significantly reduce downtime.
- Client
- Oil and Gas company
- Service
- Digital Twin, Anomaly Detection, Machine Learning
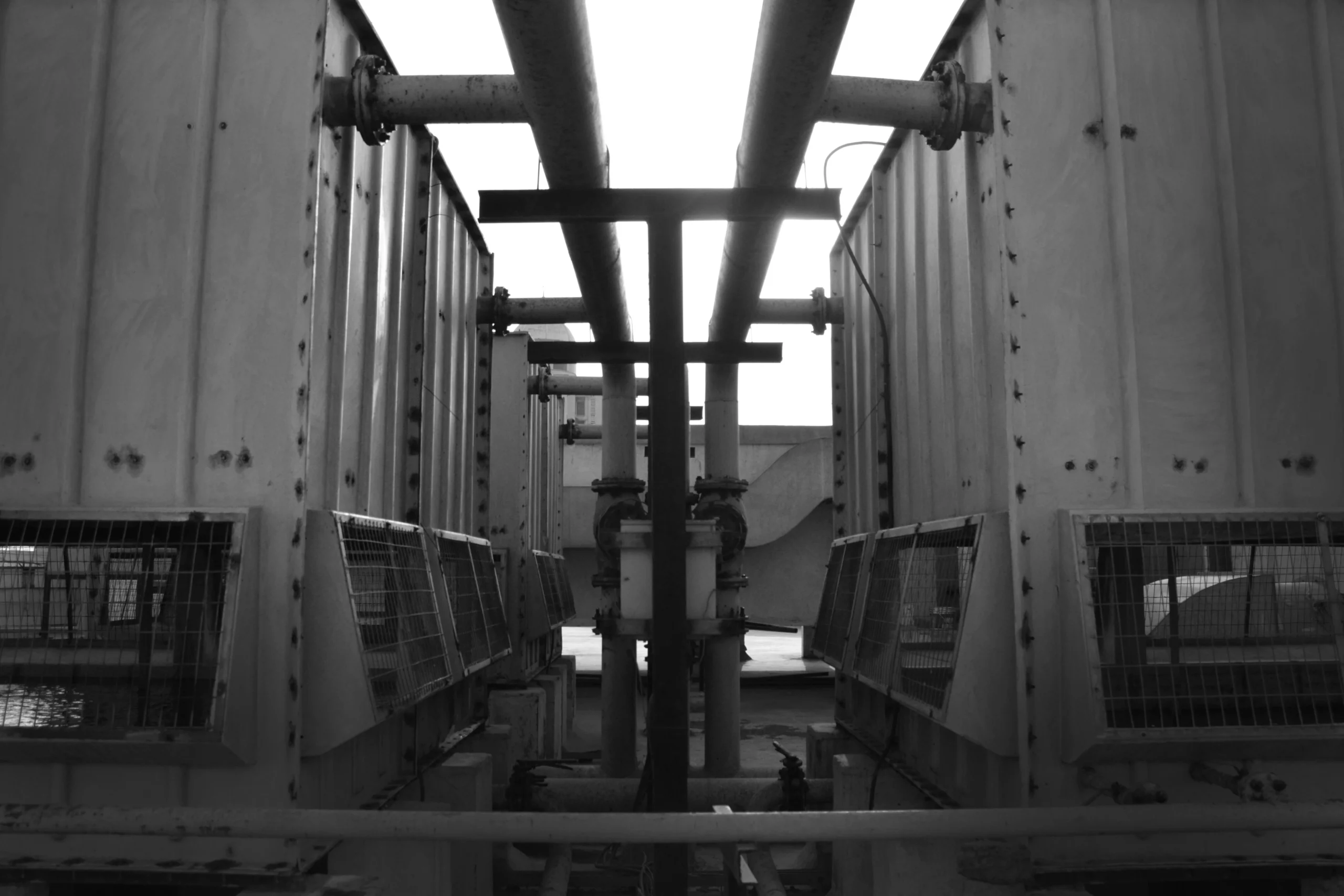
Overview
An ONGC company operated a high-value machine that experienced frequent breakdowns due to heavy usage.
The client sought to develop a digital twin for this machine to enable real-time monitoring for anomalies.
Additionally, the system needed to accurately predict potential breakdowns with a high degree of certainty, while minimizing the number of false positives to nearly zero.
What we did
We collaborated with the client to develop a proprietary machine learning algorithm for detecting anomalies in unstructured data.
We created a real-time anomaly detection platform that integrated with IoT sensors to gather data from the machine and other systems.
The platform also factored in additional variable parameters, such as weather, temperature, and utilization.
The system was designed as a self-learning model based on a continuous feedback loop.
Impact
False positives reduced at 75% from the previous implementation.
Reduction in downtime by 59%
Replicated the same setup in 2 additional plants
- Digital Twin
- Machine Learning
- Real-time systems
- Fewer False Positives
- 75%
- Reduction in downtime
- 59%